Natural Encoders occur throughout organismic and non-organismic systems in nature.
There are many kinds of artefactual, or artificial, encoders used to implement transmitters and receivers for both digital and analogue (and combined digital-analogue) machine-mediated human communication and television systems. Such encoders usually use transducers that convert one kind of energy in one medium to energy in another kind of medium. The structure of the pre-encoder source is thus converted and partially preserved and represented in a signal in a different form in the transmission medium.
Well known basic examples include transducers in older analogue telephones that convert sound waves to electrical pulses of different frequencies and wavelengths. More contemporary smartphones couple such transducers with analogue to digital converters (ADCs) to produce a digital-discrete pulse signal in the transmission medium.
It’s noteworthy that, in artefactual encoders, transducer-based encoding reduces to the physical properties of the various media employed and the natural nomic constraints or laws of physics that govern them. Thus, artefactual encoders and their human-designed transducing and modulating functions rely upon, and are constrained by, physical properties of the natural media from which they are constructed.
In nature, evolution too has produced transducer-based encoders. The peripheral and central nervous systems of animals rely on them comprehensively. All sensory modalities in mammals require receptor cells with transducers of some kind. There are different transducing receptor cells for audition (hearing), vision, somatosensation (touch, motion, and body position and image), vestibular sensation (balance), nociception (pain), and olefaction (smell).
Use of all sensory modalities involves transduction of energy from some form of proximal and distal physical stimuli into electrochemical nerve signals. Vision converts and encodes from structured electromagnetic radiation. Olefaction and gustatation convert from chemical contact. Somatosensation and nociception convert and encode from chemical and mechanical force. Audition converts and encodes from structured air pressure waves.
In addition to these sensory stimuli to nerve signal transductions, neurons themselves deploy transduction-based encoding in synaptic firing. Action potentials are converted to and from chemical and ion stored energy. Indeed, in neuroscience and psychology, the conversion of sensory stimuli to perceptual and cognitive information, or messages/structures that can be stored within and manipulated by the brain-mind, is referred to as coding.
In machine-mediated artefactual communication systems, signals generated by source/sender-transmitter couplings operate according to a set of codes for mapping source states to signal and channel states (Shannon, 1948, pp. 1–4). These codes correspond to the set of possible states of the physical information source, which is any physical stochastic process of any level of complexity from which message bearing signals can be generated and encoded. Example sources include a natural language speaker speaking, and a scene in a spatiotemporal region being captured by a camera. Moreover, since any physical stochastic process can be considered, or modelled as, a source: each process in the chain of communication can be considered a source, including the transmitter-encoder coupling.
The function of codes for such sources is to govern and track what signal forms and messages are converted from specific source states (or, in many cases, sequences of source states). The process of encoding signals and messages in the channel and transmission medium from source processes and their states is thus governed and directed by sets of codes. These sets of codes map the probability space of possible source states and sequences of source states to a channel signal space. The source states can be continuous and analogue or discrete. However, in machine mediated communication systems discrete mathematical models are usually used to simplify the design and implementation of encoders.
There are also codes for signal encoding in nature. These codes, like the encoding processes that include them, are not artefactual. They have instead evolved by natural selection and adaptation operating over hundreds of thousands to millions of years. They generally tend to be analogue and continuous, but can also have distinct identifiable discrete features such as energy peaks, thresholds in magnitudes, bandwidth limits, and remarkable spatiotemporal and dynamical features.
In signalling systems in nature, such as those involved in the sensory modalities of the nervous systems of animals, the encoding codes thus occur naturally. However, from the perspective of Shannonian, or classical, communication theory, such codes can still be modelled as being associated with natural sources and source states and their dynamics, structures, and properties.
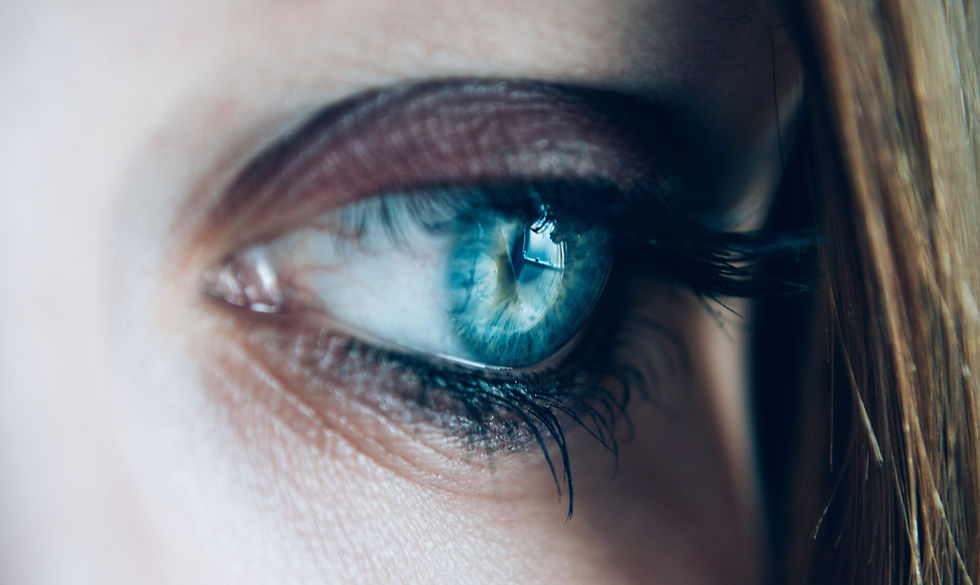
“All forms of communications employ a modulation scheme, that maps the source information onto a modulation scheme’s signal constellation.” (Tee & Taylor, 2018)
In the above source quotation, the modulation scheme corresponds with “coding scheme” or “encoding scheme, and the mapping described is essentially a characterisation of encoding. The signal constellation is the possibility space of signals, or the possible structures of signals (or of emissions) that the medium and natural channel(s) can sustain or support.
In the case of visual processing of electromagnetic and photonic sensory stimuli, there is a certain range of frequencies and wavelengths of light that can be converted into a certain bandwidth and set of nerve signals. In the case of auditory processing, a given species of animal only has the ability to sense a certain range of sound-to-auditory wavelengths. Similarly for the other sense modalities (El et al., 2015; Fay, 1984; Meister, 1996).
There are also non-organismic natural systems and processes in nature to which the natural encoding and codes model (as well as more complex developments thereof) can be applied (Adriaans, 2009, pp. 652–662). For example, astronomers commonly effectively rely upon natural codes and encoding schemes operating in cosmological and celestial phenomena that produce various kinds of X-Ray and electromagnetic emissions (Aab et al., 2014; Barrau et al., 2014; Manchester, 2004; Thornton et al., 2013).
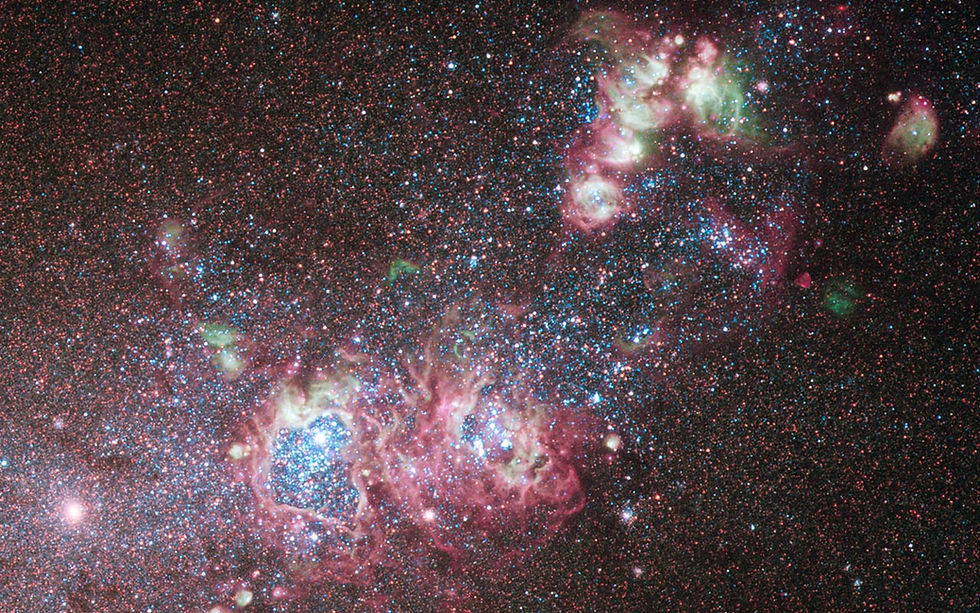
The physical processes of quasars and pulsars have specific sets of source states commensurate with their size, energy levels, natural kind designations, and internal properties and dynamics. The emissions – or signals - that they generate can be modelled as having been naturally encoded from the continuous states of the (source) processes that constitute them. The natural codes involved are realised by the physical properties and dynamics of the natural transducing processes that generate the radiated emissions from various kinds of source energy (heat, internal radiation, dynamical processes) (Abbott et al., 2008 - See especially p. 1 and 3 Re. emission mechanics and constraints; Alford & Schwenzer, 2015; Chaty, 2013).
Thus celestial emissions have effective ‘signal constellations’ or possibility spaces of signals, and their sources have effective probability spaces of source states. Natural forces operating in and around the source phenomenon enact transduction-based encoding of emissions/signals. There is a naturally occurring set of codes realised by the natural nomic constraints and physical properties of the system as a source.
Both evolved organismic and non-organismic natural encoding processes are ubiquitous. The latter - more so (ubiquitous in the known universe). They provide a way to demonstrate the elegance and power of Shannon's mathematical theory of communications. The Shannonian modelling of non-organismic celestial emission sources in cosmology can be elegantly applied - in basic terms - by treating them as Shannon sources (stochastic physical processes) and by regarding requisite codes as emission/transduction-constraining coding 'rules' realised by the physical nomic constraints acting upon, and within, the source phenomenon, and by the structure, dynamics, and properties of the source.
References
Aab, A., Abreu, P., Aglietta, M., Ahlers, M., Ahn, E. J., Al Samarai, I., Albuquerque, I. F. M., Allekotte, I., Allen, J., Allison, P., Almela, A., Castillo, J. A., Alvarez-Muñiz, J., Batista, R. A., Ambrosio, M., Aminaei, A., Anchordoqui, L., Andringa, S., Aramo, C., … Ziolkowski, M. (2014). A search for point sources of EeV photons. Astrophysical Journal. https://doi.org/10.1088/0004-637X/789/2/160
Abbott, B., Abbott, R., Adhikari, R., Ajith, P., Allen, B., Allen, G., Amin, R., Anderson, S. B., Anderson, W. G., Arain, M. A., Araya, M., Armandula, H., Armor, P., Aso, Y., Aston, S., Aufmuth, P., Aulbert, C., Babak, S., Ballmer, S., … Collaboration, L. S. (2008). Beating the spin-down limit on gravitational wave emission from the Crab pulsar. ASTROPHYSICAL JOURNAL LETTERS, 683(1), L45--L49.
Adriaans, P. (2009). Between order and chaos: The quest for meaningful information. Theory of Computing Systems, 45(4), 650–674. https://doi.org/10.1007/s00224-009-9173-y
Alford, M. G., & Schwenzer, K. (2015). Gravitational wave emission from oscillating millisecond pulsars. MONTHLY NOTICES OF THE ROYAL ASTRONOMICAL SOCIETY, 446(4), 3631–3641.
Barrau, A., Rovelli, C., & Vidotto, F. (2014). Fast radio bursts and white hole signals. Physical Review D - Particles, Fields, Gravitation and Cosmology. https://doi.org/10.1103/PhysRevD.90.127503
Chaty, S. (2013). Optical/infrared observations unveiling the formation, nature and evolution of High-Mass X-ray Binaries. Advances in Space Research. https://doi.org/10.1016/j.asr.2013.09.010
El, J. B., E.J., W., M.J., B., L., K., E., B., J., S., & M., D. (2015). Neural coding underlying the cue preference for celestial orientation. In Proceedings of the National Academy of Sciences of the United States of America.
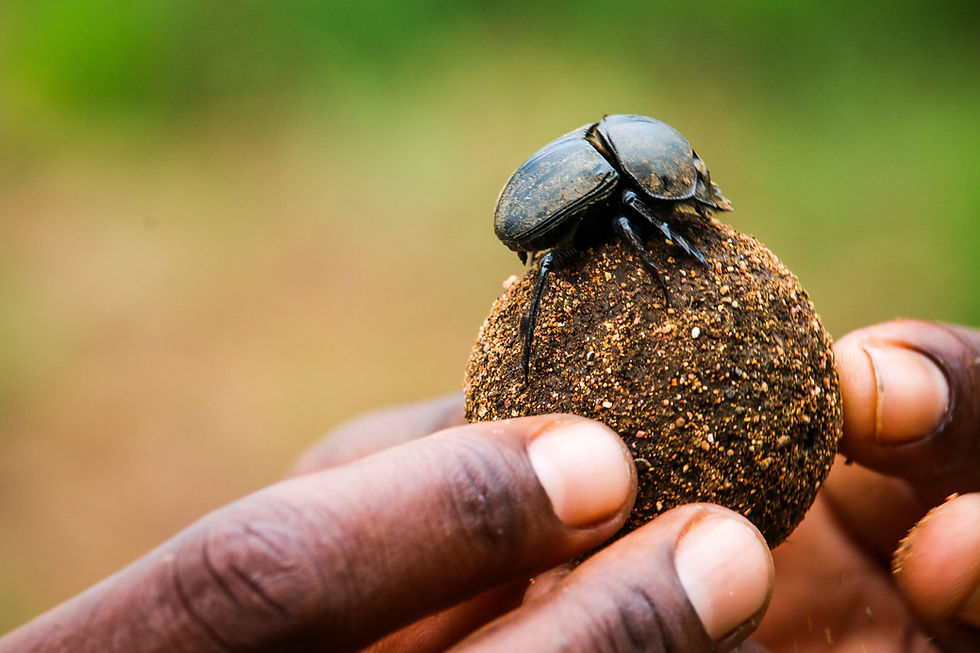
Fay, R. R. (1984). The goldfish ear codes the axis of acoustic particle motion in three dimensions. Science. https://doi.org/10.1126/science.6474161
Manchester, R. N. (2004). Observational Properties of Pulsars. In Science. https://doi.org/10.1126/science.1097649
Meister, M. (1996). Multineuronal codes in retinal signaling. Proceedings of the National Academy of Sciences of the United States of America. https://doi.org/10.1073/pnas.93.2.609
Shannon, C. E. (1948). A Mathematical Theory of Communication. Bell System Technical Journal. https://doi.org/10.1002/j.1538-7305.1948.tb01338.x
Tee, J., & Taylor, D. P. (2018). Is Information in the Brain Represented in Continuous or Discrete Form? Undefined. http://arxiv.org/abs/1805.01631
Thornton, D., Stappers, B., Bailes, M., Barsdell, B., Bates, S., Bhat, N. D. R., Burgay, M., Burke-Spolaor, S., Champion, D. J., Coster, P., D’Amico, N., Jameson, A., Johnston, S., Keith, M., Kramer, M., Levin, L., Milia, S., Ng, C., Possenti, A., & Van Straten, W. (2013). A population of fast radio bursts at cosmological distances. Science. https://doi.org/10.1126/science.1236789
Other Sources
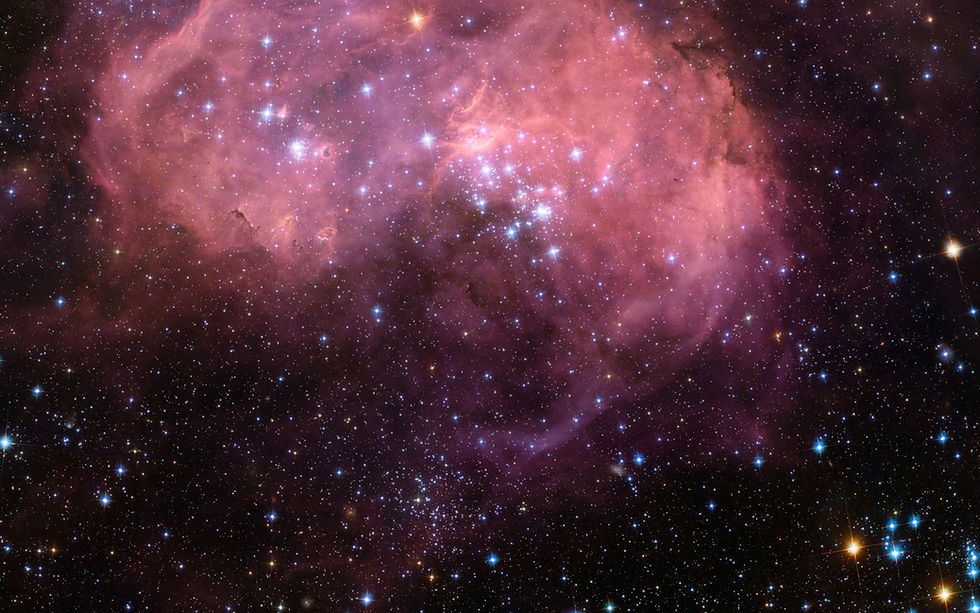
Abramowski, A., Aharonian, F., Ait Benkhali, F., Akhperjanian, A. G., Angüner, E. O., Backes, M., Balenderan, S., Balzer, A., Barnacka, A., Becherini, Y., Becker Tjus, J., Berge, D., Bernhard, S., Bernlöhr, K., Birsin, E., Biteau, J., Böttcher, M., Boisson, C., Bolmont, J., … Fukui, Y. (2014). Diffuse Galactic gamma-ray emission with H.E.S.S. Physical Review D - Particles, Fields, Gravitation and Cosmology. https://doi.org/10.1103/PhysRevD.90.122007
Adriani, O., Barbarino, G. C., Bazilevskaya, G. A., Bellotti, R., Boezio, M., Bogomolov, E. A., Bongi, M., Bonvicini, V., Bottai, S., Bruno, A., Cafagna, F., Campana, D., Carlson, P., Casolino, M., Castellini, G., Donato, C. De, Santis, C. De, Simone, N. De, Felice, V. Di, … Zampa, N. (2015). SEARCH for ANISOTROPIES in COSMIC-RAY POSITRONS DETECTED by the PAMELA EXPERIMENT. Astrophysical Journal. https://doi.org/10.1088/0004-637X/811/1/21
Andersen, R. A., Essick, G. K., & Siegel, R. M. (1985). Encoding of spatial location by posterior parietal neurons. Science. https://doi.org/10.1126/science.4048942
Barbieri, M. (2015). Code biology: A new science of life. In Code Biology: A New Science of Life. https://doi.org/10.1007/978-3-319-14535-8
Barbieri, M. (2018). What is code biology? In BioSystems. https://doi.org/10.1016/j.biosystems.2017.10.005
Barbieri, M. (2008). The codes of life. In the Rules of Macroevolution.
Barbieri, M., & Barbieri, M. (2015). Code Biology. In Code Biology. https://doi.org/10.1007/978-3-319-14535-8_10
Chaty, S. (2013). Optical/infrared observations unveiling the formation, nature and evolution of High-Mass X-ray Binaries. Advances in Space Research. https://doi.org/10.1016/j.asr.2013.09.010
D’Elia, V., Perri, M., Puccetti, S., Capalbi, M., Giommi, P., Burrows, D. N., Campana, S., Tagliaferri, G., Cusumano, G., Evans, P., Gehrels, N., Kennea, J., Moretti, A., Nousek, J. A., Osborne, J. P., Romano, P., & Stratta, G. (2013). The seven year Swift-XRT point source catalog (1SWXRT). Astronomy and Astrophysics. https://doi.org/10.1051/0004-6361/201220863
Galletti, C., Battaglini, P. P., & Fattori, P. (1993). Parietal neurons encoding spatial locations in craniotopic coordinates. Experimental Brain Research. https://doi.org/10.1007/BF00227102
Godfrey-Smith, P., & Sterelny, K. (2016). Biological Information. In E. N. Zalta (Ed.), The {Stanford} Encyclopedia of Philosophy (Summer 2016). Metaphysics Research Lab, Stanford University.
Guimarães, R. C. (2003). The Organic Codes: an introduction to semantic biology. Genetics and Molecular Biology. https://doi.org/10.1590/s1415-47572003000100017
Minderer, M., Brown, K. D., & Harvey, C. D. (2019). The Spatial Structure of Neural Encoding in Mouse Posterior Cortex during Navigation. Neuron. https://doi.org/10.1016/j.neuron.2019.01.029
Parker, S. C. J., & Tullius, T. D. (2011). DNA shape, genetic codes, and evolution. In Current Opinion in Structural Biology. https://doi.org/10.1016/j.sbi.2011.03.002
Reddy, L., & Kanwisher, N. (2007). Category Selectivity in the Ventral Visual Pathway Confers Robustness to Clutter and Diverted Attention. Current Biology. https://doi.org/10.1016/j.cub.2007.10.043
Sterelny, K. (2000). The “Genetic Program” Program: A Commentary on Maynard Smith on Information in Biology. Philosophy of Science. https://doi.org/10.1086/392769
van Boxtel, J. J. A., Alais, D., & Van Ee, R. (2008). Retinotopic and non-retinotopic stimulus encoding in binocular rivalry and the involvement of feedback. Journal of Vision. https://doi.org/10.1167/8.5.17
Weeks, K. M., & Mauger, D. M. (2011). Exploring RNA structural codes with SHAPE chemistry. Accounts of Chemical Research. https://doi.org/10.1021/ar200051h
Wenderoth, P., & Wiese, M. (2008). Retinotopic encoding of the direction aftereffect. Vision Research. https://doi.org/10.1016/j.visres.2008.06.013
Zylberberg, J., Pouget, A., Latham, P. E., & Shea-Brown, E. (2017). Robust information propagation through noisy neural circuits. PLoS Computational Biology. https://doi.org/10.1371/journal.pcbi.1005497
Commentaires